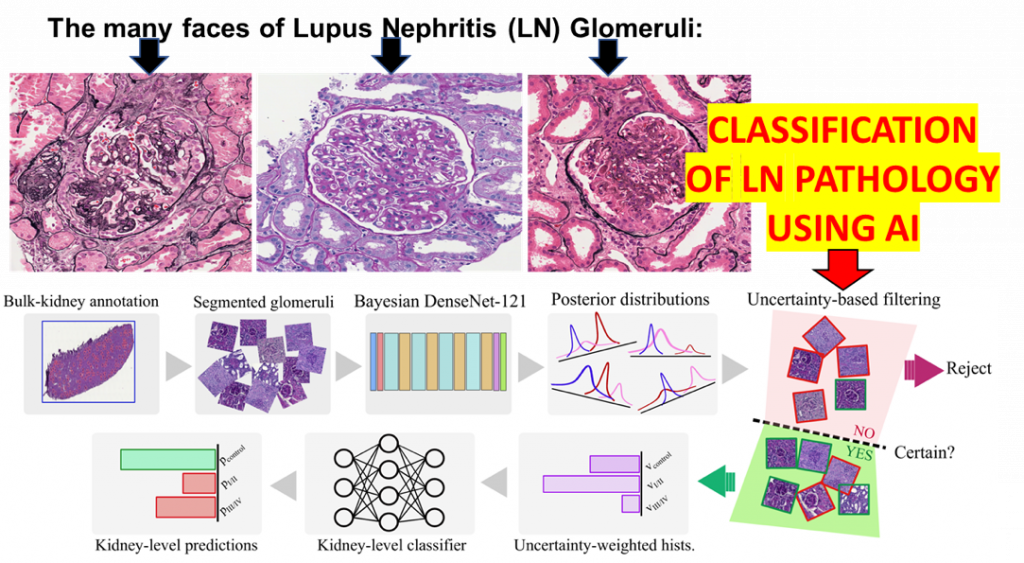
Can AI help classify lupus nephritis? Convolutional Neural Networks for classification of murine and human LN
Lead: Pietro Antonio Cicalese
Co-mentor: Dr. Hien Van Nguyen
Collaborators: Drs. Chang, Truong, Becker, Sadhna, Khambam, Torrealba
Project Summary:
Our group is interested in developing a Computer Vision (CV) pipeline for the classification of Lupus Nephritis (LN) with histopathology imaging data. Our objective is to leverage the power of Deep Neural Network (DNN) architectures to create an effective Computer Aided Diagnosis (CAD) system that physicians can then use to supplement their diagnosis. Eventually, we would like to develop stand-alone systems that can be used as an alternative to the physician or pathologist, for diagnosing the disease.
What is already known in the field?
There are very few groups working with LN data in this context. While some binary and ternary classifiers have been developed, our group is at the forefront of this technology in terms of the precision of our proposed classification schemes.
What is new?
Our group has the advantage of having both solid domain knowledge and technical knowhow in the field of CV; we always implement the most powerful (and recent) architectures and constantly work to develop novel architectures that custom fit our task. We are also working to develop classifiers that can describe each morphological feature to then classify disease.
Why is this important?
Given the complex nature of LN, disagreement surrounding the morphology of the disease, and the many scoring parameters used to assess LN glomeruli, physicians and pathologists struggle to consistently reproduce their diagnosis across labs. This is indeed a significant problem because how the LN patient is treated depends on the pathologist’s diagnosis. This problem can be solved with AI; utilizing consensus scores and changing how we think about classification provide us with an exciting research direction that can one day help physicians improve the quality of the care they provide.
Ongoing/future steps:
We are investigating multiple different solutions to this problem, ranging from segmentation and supervised classification to fully unsupervised methods. We are currently cataloging a large bank of LN kidney biopsy slides that are being annotated by multiple nephropathologists which we then intend to make public once fully curated, so that these may drive AI-based diagnostics of LN. Similar approaches are also being extended to related renal diseases.